Effects of AVs on parking choice
Student information
Author: Daphne Elisabeth Maria van den Hurk
Institution: Delft University of Technology
Graduation year: 2017
An empirical study into the effects of private automated vehicles on motorists’ parking location choice: an application to the city of The Hague
Automated vehicles (AVs) have been receiving increased attention all over the world, since the first fully AVs are already operating on the public road network. AVs could not only have a tremendous impact on the urban environment but also on human travel behaviour. With the capability of AVs to ride and park themselves instead of being operated by a human driver, it is likely that parking choice behaviour will change when conventional vehicles (CVs) are replaced by AVs. In order to make investment decisions, it is important for governments to gain insight into the impacts of AVs. The objective of this research is to find the importance of different factors and constraints that could influence drivers’ parking location choice for a future situation in which private highly AVs will become available for passenger transport. The results of this study have been used to provide guidelines for governments on how to develop their parking policy for this future situation. The main research question of this thesis is formulated as follows:
‘’ What is the effect of private highly automated vehicles on drivers’ parking location choice, based on parking constraints? ’’
AVs can either be privately used or shared with others. This research is focused on the private use of AVs. A schematic overview of a trip with a private highly AV is visualised in Figure 1. The trip with a private highly AV starts from the ‘passenger origin’ and develops in the direction of the ‘passenger destination’. Space to drop-off the passenger is needed to avoid congestion caused by dropping-off passengers on the road itself. On-street parking space is used for the drop-off manoeuvre. When the passenger is dropped-off at a drop-off point, the passenger walks to the destination.
Figure 1 Schematic overview of the different steps of a trip with a private highly AV
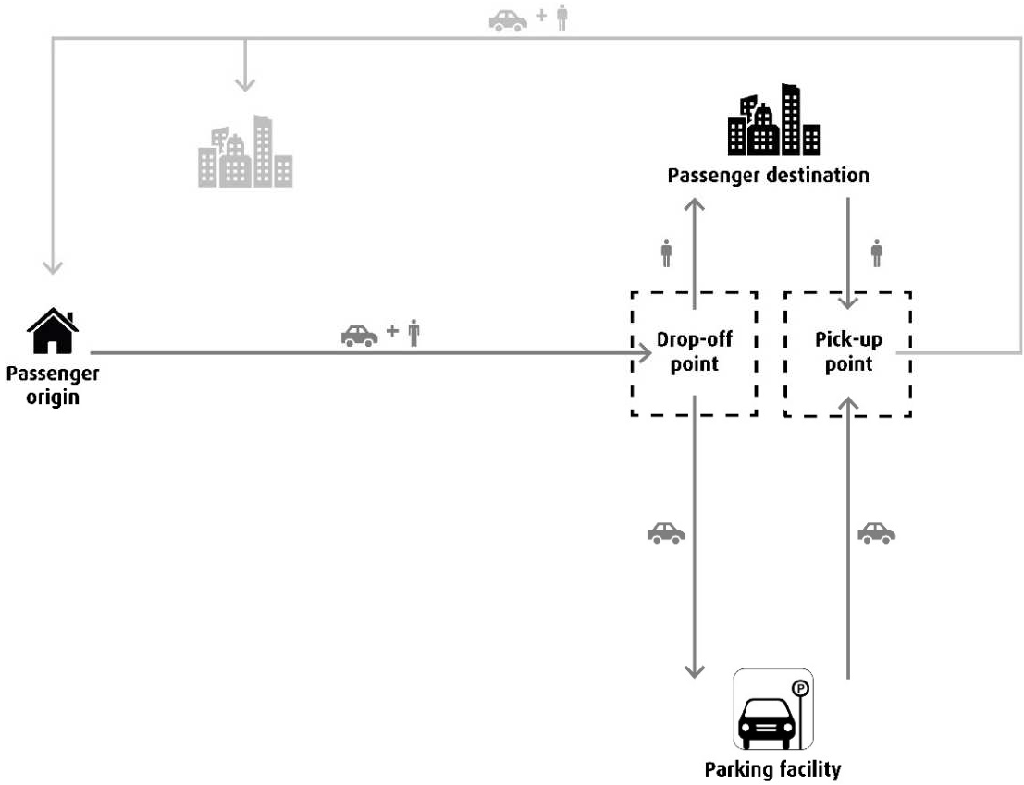
Simultaneous to this walking leg, the private highly AV drives empty from the drop-off point to a parking facility. The two considered parking locations are 1) parking in the inner city (PIC) and 2) parking at the edge of the city (PEC), both at off-street parking facilities. When the passenger’s activity has ended, he/she walks to a pick-up point. On-street parking space is used for the pick-up manoeuvre. Simultaneously, the private highly AV drives empty from the parking facility to the pick-up point. When the passenger and the private highly AV have both arrived at the pick-up point, the vehicle trip from the pick-up point to the passenger’s home or to another destination starts.
A literature review and brainstorm sessions with experts were conducted to define factors and constraints that could influence drivers’ parking location choice. Factors and constraints for the Stated Preference (SP) experiment were selected by means of a Multi-Criteria Analysis (MCA). The selected factors and constraints can be divided into different categories: context factors, attributes, perceptions and exogenous variables. A SP data collection method was used in this research to examine which factors and constraints, and to which extent, influence a driver’s parking location choice. Private highly AVs as described in this study are not operating on the public road network yet, which makes the need for hypothetical choice situations necessary. SP data is based on individuals’ reactions to hypothetical situations: it is asked what an individual would choose in a specific situation. In this research the environmental conditions, road network configuration and parking constraints of the city of The Hague are used specifically, however, the generic methodology applied in this study could be applied to any large scale city.
Two pilot surveys were conducted in order to design the final questionnaire. An orthogonal design was used to create the hypothetical choice situations for both pilot surveys, because there is no information on prior parameter values. The aim of both pilot surveys was to test if the respondents understood the questionnaire and the concept of automated driving. Furthermore, the results of both pilot surveys were used to find prior parameter values. A final survey was made, based on the results of both pilot surveys. The final survey consists of introduction questions, hypothetical choice situations (part 1), statements on automated driving (part 2) and general questions on personal characteristics (part 3).
In the introduction questions, respondents’ fill in the trip characteristics (trip purpose, trip duration and trip reimbursement) of their most recent trip to the inner city of The Hague. The trip characteristics are the context factors that apply for the hypothetical choice situations which were asked in the first part of the survey. Preferences regarding the attributes were collected via the different hypothetical choice situations. Attributes included in the design are: ‘parking cost’, ‘surveillance of the parking facility’, ‘risk of extra waiting time’ and ‘risk of parking fee’. The two latter attributes are new concepts for individuals, describing respectively the result of the vehicle arriving too early at the pickup point and the vehicle arriving too late at the pick-up point. An efficient design was used to create the hypothetical choice situations, because the pilot survey provided information on the prior parameter values. In the second part of the survey, statements were presented in order to receive information on respondents’ perceptions on automated driving. Information about respondents’ exogenous factors was collected via general questions in the third part of the survey.
In total, 421 respondents filled in the online questionnaire. 388 responses were valid and used for the data analysis. Results of the descriptive analysis showed that 16.2% of the respondents have a fixed preference for PIC, compared to 11.6% of the respondents that have a fixed preference for PEC. Trip characteristics explain the fixed preference for either PIC or PEC. Results of the Multinomial logit (MNL) model estimation on the hypothetical choice situations show that all attributes are significant, which means that these attributes are of influence on drivers’ parking location choice. From the results of the hypothetical choice situations, it can be concluded that in general PIC is preferred over PEC. The ‘parking cost’, the ‘risk of extra waiting time’ and the ‘risk of parking fee’ have a negative influence on drivers’ parking location choice. ‘Personnel surveillance’ has a positive influence on drivers’ parking location choice. The parameter for ‘camera surveillance’ is not significant, which means that individuals are not sensitive for the presence of cameras in a parking facility. Personal characteristics (exogenous factors), trip characteristics (context factors) and perceptions resulting from the MCA were included in the MNL model as interaction effects to test if these characteristics affect the attributes that influence drivers’ parking location choice. Results of the MNL model estimation on the interaction effects showed that only a few interaction effects are significant. Despite their significance, several of these interaction effects are based on a small sample and others cannot be explained. The following interaction effects are based on a large sample and can be explained:
Individuals with a high income are more sensitive for ‘risk of extra waiting time’. This was expected, since the research pointed out that on average, individuals with a higher income have a higher Value of Time (VoT) and Value of Reliability (VoR).
Individuals with a relatively high purchase value of the car are less sensitive for ‘risk of extra waiting time’. A reason for this might be that individuals with a high purchase value of the car find it more important that the car arrives safely at the passenger’s destination. In this case, the individual accepts the ‘risk of extra waiting time’.
Individuals who consider safety during the empty vehicle trip to be important, are less sensitive for the ‘risk of extra waiting time’ and the ‘risk of parking fee’. Apparently, these individuals care more about the safety circumstances during the empty vehicle trip than about extra time and costs.
When a large amount of interaction effects do not play a role, a more generic model can be estimated that works for the same conditions. Therefore, it was chosen to conduct the scenario analysis based on a model without interaction variables. This means that the same model applies for individuals with different characteristics, trip purposes and perceptions.
The results of the scenario analysis are visualised in Figure 2. From the results of the scenario analysis can be concluded that individuals are most sensitive for a change in direct costs, i.e. the ‘parking cost’ at the parking facility and the ‘parking fee’ for temporary parking the highly AV at an on-street parking place near the passenger’s destination. When the parking cost in the inner city is decreased with €1 per hour, parking demand will increase with 11%. Furthermore, it could be expected that when the parking cost in the inner city will be increased with €1 per hour, parking demand will decrease with 8%. When there are no parking costs for parking at the edge of the city, parking demand will remain the same. When the parking cost at the edge of the city will be increased from €4 per day to €8 per day or €12 per day, it is expected that parking demand will drastically decrease with 15% and 45% respectively. When a parking fee of €20 is implemented for temporary parking the highly AV at an on-street parking place near the passenger’s destination, parking demand at the edge of the city will decrease with 19%. This has the same effect as increasing the parking cost at the edge of the city from €4 to approximately €8.50 per day. From the results of the scenario analysis can be concluded that individuals are less sensitive for ‘personnel surveillance’ and ‘risk of extra waiting time’. The presence of personnel surveillance has a positive influence on drivers’ parking location choice. When personnel surveillance will be available at a parking facility, parking demand will increase with 6% in the inner city, compared to 3% at the edge of the city. From the results of the model, it was concluded that camera surveillance is not significant, which means that camera surveillance is valued the same as no surveillance. This means that when the parking facility is supervised by means of cameras, it is expected that this will not lead to an increase or decrease in parking demand. The risk of extra waiting time (for 10 minutes) during the off-peak period is 1 out of 10 times. When no separated lanes for highly AVs exist, the risk of extra waiting time during the peak period is likely to be higher. When the risk of extra waiting time is increased to 3 out of 10 times or 5 out of 10 times during the peak period, and no separated lanes for highly AVs are available, the parking demand at the edge of the city will decrease to 5% and 9% respectively.
Figure 2 The influence of the what-if scenarios on the distribution of parking demand
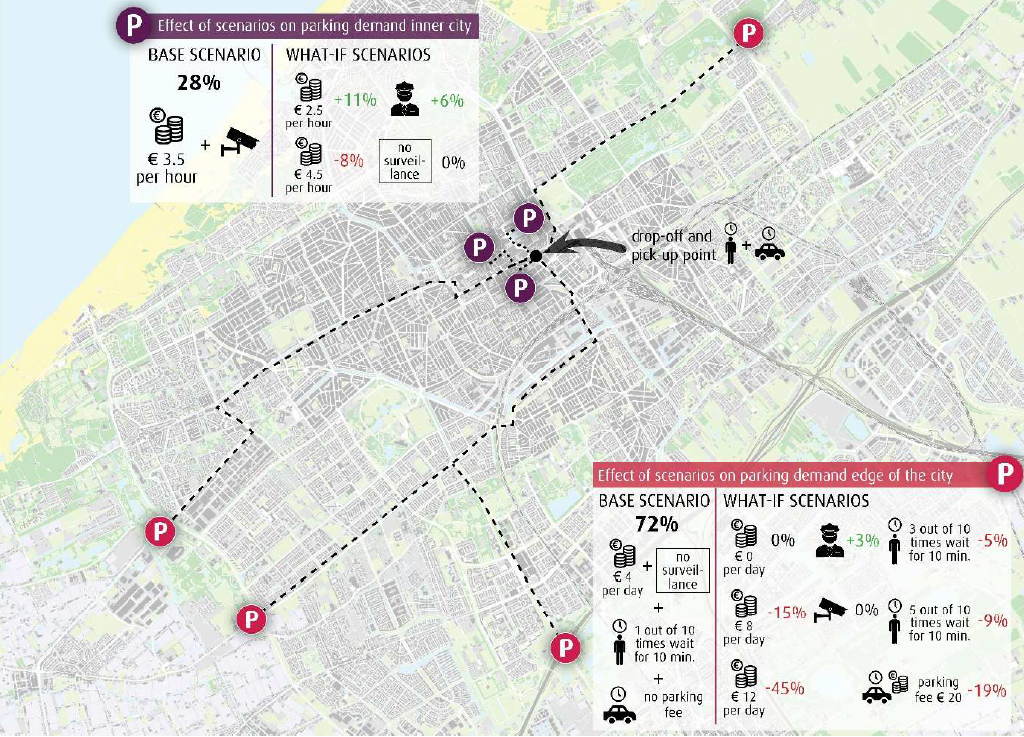
Directions for parking policies are related to different topics regarding parking regime, parking price and parking capacity. The directions for parking policies are visualised in Figure 3.
First, in order to reduce the number of on-street parking spaces, it is advised to forbid the parking of highly AVs at on-street parking spaces. Consequently, released space could be used for drop-off and pick-up manoeuvres. It is not expected that all on-street parking space is needed for drop-off and pick-up manoeuvres. Similar to the current situation, it might be considered that inhabitants of the city of The Hague are allowed to park their highly AV on-street with a parking permit. Furthermore, released on-street parking space could be used for greenery or extra space for bicyclists and pedestrians.
Second, in order to minimize the number of empty vehicle kilometres, it is advised to stimulate short term parking of highly AVs in the inner city and stimulate long term parking of highly AVs at the edge of the city. This could be done by increasing the parking cost of parking at the edge of the city from €4 to €10 per day. Consequently, approximately 55% of the individuals would park their highly AV in the inner city, compared to 28% that parked their highly AV in the inner city in the base scenario.
Third, it is advised to implement a dynamic pricing strategy for the parking fee that is asked for temporary parking the highly AV at an on street parking place near the passenger’s destination, when the highly AV arrives too early. When implementing a dynamic pricing strategy, the municipality is able to 1) control supply and demand, 2) account for competitor pricing and 3) account for external factors (e.g. peak periods). When a parking fee of €20 is implemented, approximately 47% of the individuals would park their highly AV in the inner city, compared to 28% that parked their highly AV in the inner city in the base scenario. Fourth, when more parking capacity is needed, it is advised to invest in flexible parking facilities at the edge of the city near distributor roads. When the parking facility is supervised by personnel, parking demand will only increase with 3%. To increase the attractiveness of parking highly AVs at the edge of the city, it is advised to reserve space for additional services (e.g. pick-up point for groceries and day-care).
Further research is needed to examine which services positively influence drivers’ parking location choice. Recent studies show that automated vehicles could induce an increase of travel demand due to changes in destination choice, mode choice and mobility (Milakis, Arem, & Wee, 2017). Hence, more parking capacity might be required. Furthermore, the level of sharing and the penetration rate of AVs should be taken into account when making policy decisions, because these developments might have an influence on the number of parking spaces required. This research succeeded in capturing the change of drivers’ parking location choice in the case when private highly AVs will become available for passenger transport. As a result of choices made by respondents in the hypothetical choice situations, insight was gained in individuals’ preferences and trade-offs. The presented results and guidelines can be used in future research on the effects of highly AVs on parking location choice where, at the same time, it can be used by governments to develop their parking policy for this future situation.
Figure 3 Visualisation of the directions for promising parking policies
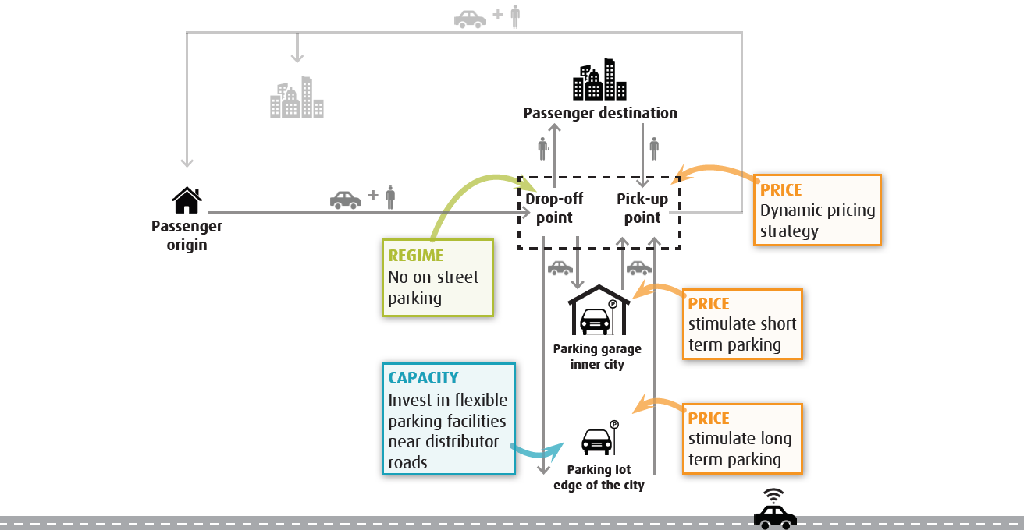