Drop zone locations
Student information
Author: Evi Rombouts
Institution: University of Antwerp
Graduation year: 2024
Finding suitable drop zone locations for free-floating forms of micromobility
Over the past decade, shared scooters have become increasingly visible on the urban streetscape, complementing traditional means of transport such as shared bicycles and public transport. Thanks to their eco-friendliness, limited spatial impact and constant availability, shared scooters can contribute to urban traffic, particularly through their potential to reduce the number of cars in the city. Although shared scooters are currently mainly used recreationally by young, affluent men, there are opportunities to encourage their use and realise the full potential of this service.
However, there are some problems with shared scooters that negatively affect public opinion. A key problem is that shared scooters are often parked in a way that blocks the pavement, as they are free-floating and can be left anywhere. This research focuses on solving this problem by locating drop zones so that the scooters can become station-based, without users having to walk long distances to their destination.
Research methods
To localise these drop zones, two methods were used in this research: the unsupervised learning method k-means clustering and the optimisation model MCLP (Maximal Coverage Location Problem). Both methods have their own strengths. K-means clustering performs better in terms of mean and median distances, meaning users on average have to walk less far to reach a shared scooter. MCLP, on the other hand, maximises coverage, serving a larger percentage of demand within a 200-metre radius.
Comparison of K-means clustering and MCLP
As the number of stops increases, the performance of k-means clustering and MCLP start to come closer together, especially in terms of coverage ratio. This suggests that with enough stops, the choice between the two methods becomes less crucial. K-means clustering tends to generate a balanced distribution of stops throughout the study area, resulting in lower average distances. MCLP, on the other hand, distributes stops more towards the centre where demand is concentrated, which can be advantageous in urban centres with high demand. A disadvantage of MCLP is that it neglects the suburbs and the capacity of stops can still be high.
A possible improvement for MCLP is to implement a capacitated MCLP, setting a maximum capacity per stop as an additional constraint. However, this could lead to a higher concentration of stops at congested locations such as near the central station, which requires a larger number of stops to achieve the same coverage ratio.
K-means clustering can be affected by the random initial point distribution, which can lead to variable results. To improve k-means clustering performance, the method can be run multiple times to compare different local optima. Besides performance indicators such as distance and coverage ratio, practical aspects including the workload for providers to redistribute and possible pavement blockages should also be considered. Moreover, adjustments such as moving stops to wider locations may be necessary, which may slightly worsen performance indicators.
Evaluation of mobility hubs
The evaluation of mobility hubs based on MCLP and k-means clustering logically shows that the introduction of these hubs does not lead to improvements in walking distance and availability of drop zones within a 200-metre radius.
For MCLP mobility hubs, performance indicators for a 50-metre radius are almost identical to those for 100 meters, probably due to the impact of high demand drop zones in the city centre, such as at the Central Station and Groenplaats. Small shifts in those busy zones have a big impact on performance. MCLP uses a grid to concentrate demand in specific points. Small shifts in drop zone location can cause these points to fall outside the coverage radius, which can significantly reduce performance. As a result, the coverage ratio of MCLP mobility hubs remains low compared to that of k-means clustering hubs making it recommended to use a different method if one would like to use the current public transport network for locating shared scooter stations.
K-means clustering distributes drop zones more evenly across the city, even with increasing values of k, allowing neighbouring drop zones to absorb demand when shifts occur and reducing the impact of changes. Therefore, in the case of k-means clustering, one could conclude that the creation of mobility hubs does add value.
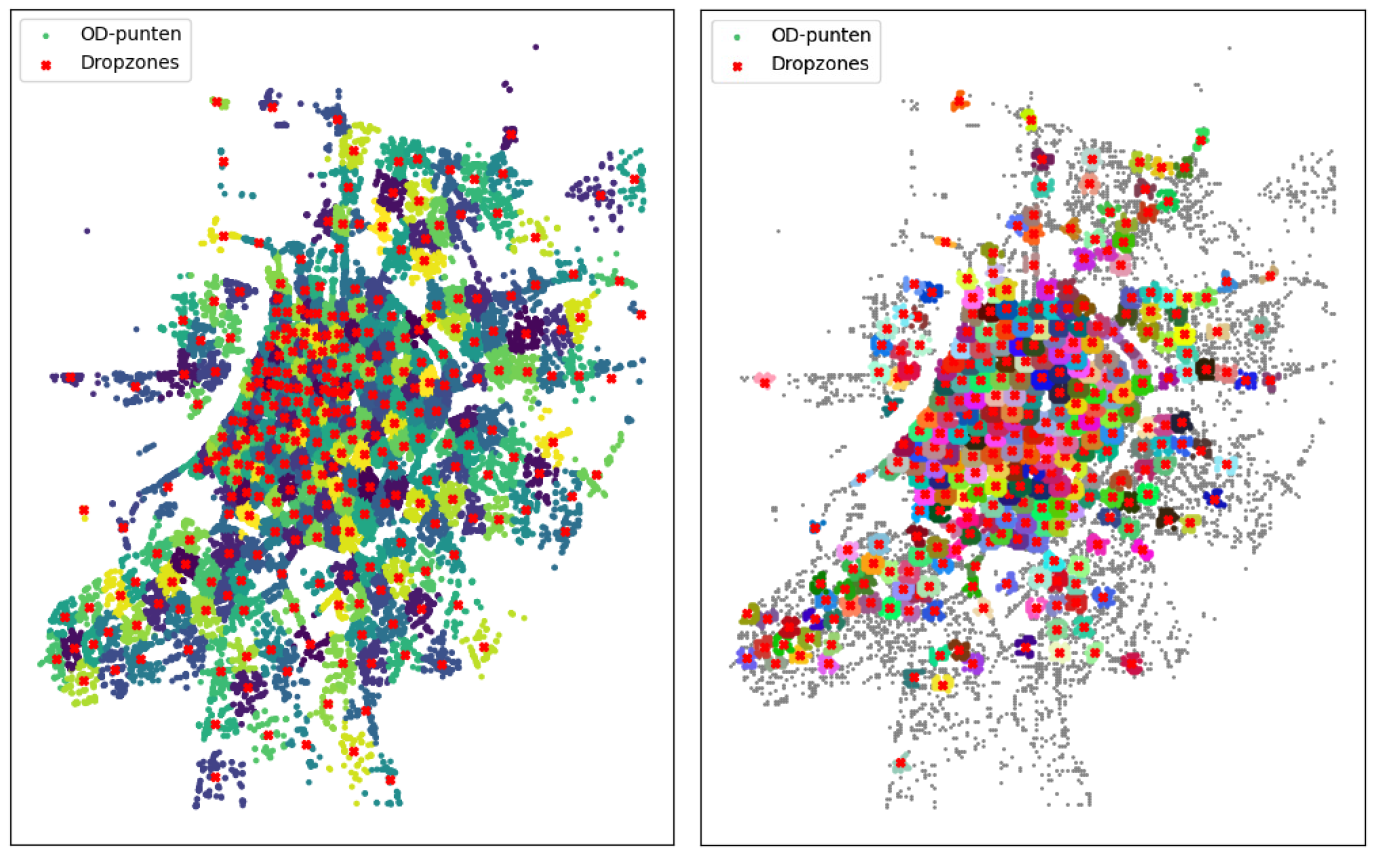
K-means clustering (k=300) and MCLP (n-300) results
Recommendations
These study results could be used by the city of Antwerp to require providers of free-floating shared scooters to use designated drop zones. The choice of method and optimal number of stops depends on the city authorities' interests and objectives. However, the recommendation is to implement more than 100 stops, as the results for this number are significantly worse than for larger numbers.
Limitations of the Study
Note that this study was conducted with data from only one of the three shared scooter providers in the city. While the dynamics of other providers are expected to be similar, it may be relevant to include them all in the clustering or optimisation exercise. In addition, required drop zone capacity is currently based only on one provider's fleet. Another limitation is that only data from the month of June was used. Other months may show different trends, as use of shared scooters can be affected by seasonal influences such as weather.